Improving Intrusion Detection Systems Using Zero-Shot Recognition Via Graph Embeddings
2020 IEEE 44TH ANNUAL COMPUTERS, SOFTWARE, AND APPLICATIONS CONFERENCE (COMPSAC 2020)(2020)
摘要
In order to detect insider threats, anomaly-based intrusion detection must learn profiles of normal user behavior. However this is particularly difficult when historical audit data is scarce. Zero-shot learning can address this limitation by compensating the absence of examples with semantic knowledge, allowing to better estimate behavior of unknown users. In this paper, we address insider threat detection in two use cases where historical user data is unavailable or obsolete. We extend an existing intrusion detection system by adding information describing user positions, roles and projects assignments. These semantic descriptions are encoded via graph embeddings. Experimental results show that providing this additional context improves insider threat detection significantly. This suggests that zero-shot learning is a promising way of improving intrusion detection systems.
更多查看译文
关键词
zero-shot learning, intrusion detection system, graph embedding, security, anomaly detection
AI 理解论文
溯源树
样例
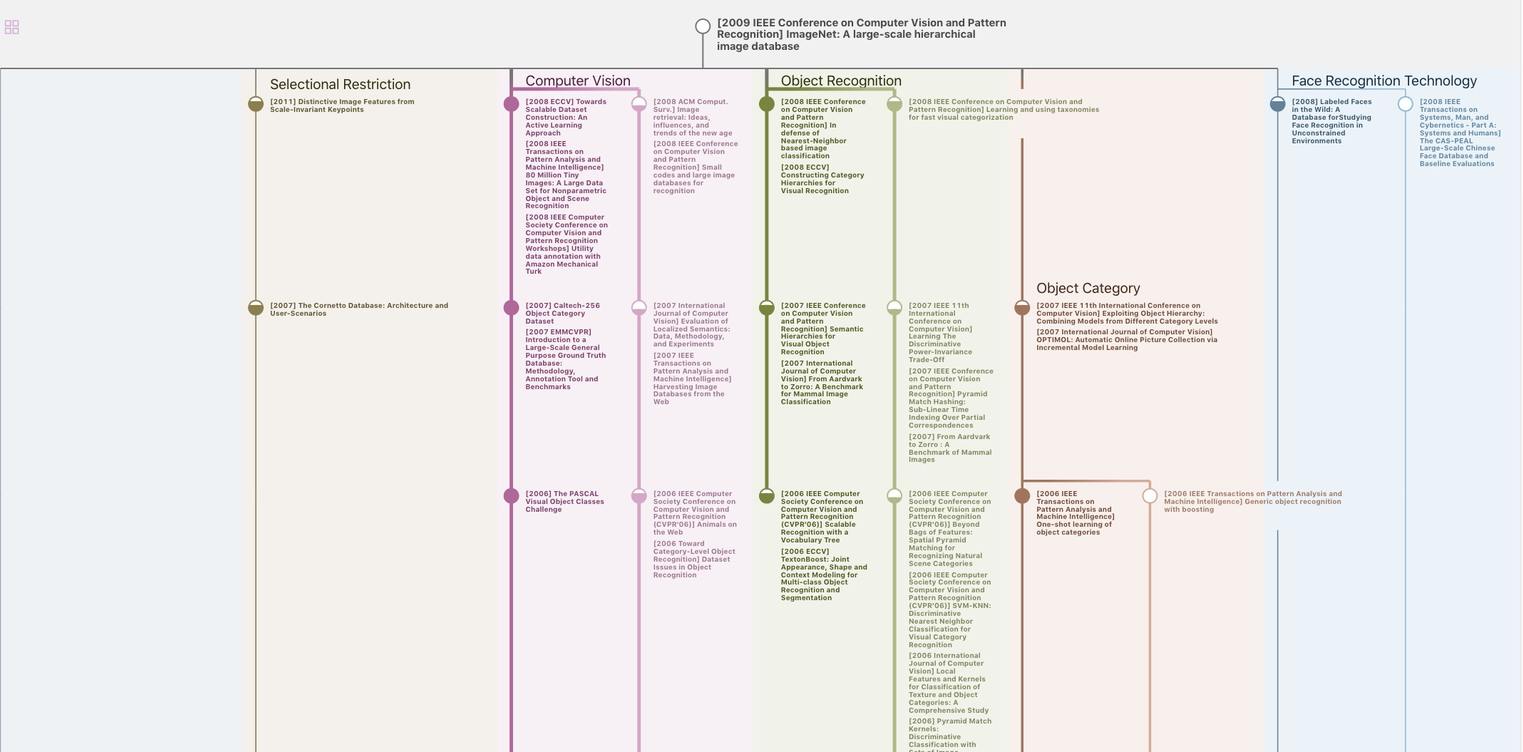
生成溯源树,研究论文发展脉络
Chat Paper
正在生成论文摘要