Bootstrapped nDCG Estimation in the Presence of Unjudged Documents.
ECIR (1)(2023)
摘要
Retrieval studies often reuse TREC collections after the corresponding tracks have passed. Yet, a fair evaluation of new systems that retrieve documents outside the original judgment pool is not straightforward. Two common ways of dealing with unjudged documents are to remove them from a ranking (condensed lists), or to treat them as non- or highly relevant (naïve lower and upper bounds). However, condensed list-based measures often overestimate the effectiveness of a system, and naïve bounds are often very “loose”—especially for nDCG when some top-ranked documents are unjudged. As a new alternative, we employ bootstrapping to generate a distribution of nDCG scores by sampling judgments for the unjudged documents using run-based and/or pool-based priors. Our evaluation on four TREC collections with real and simulated cases of unjudged documents shows that bootstrapped nDCG scores yield more accurate predictions than condensed lists, and that they are able to strongly tighten upper bounds at a negligible loss of accuracy.
更多查看译文
AI 理解论文
溯源树
样例
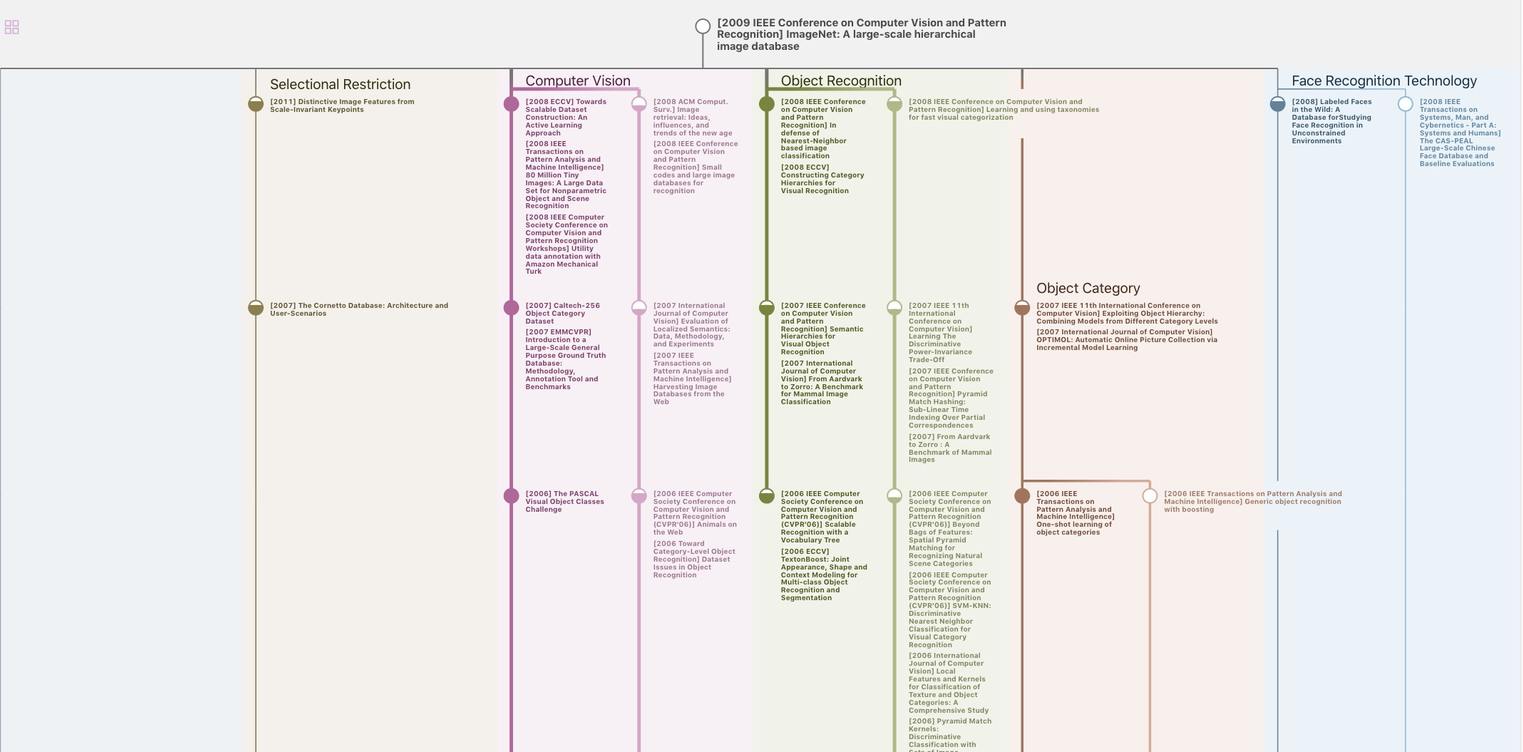
生成溯源树,研究论文发展脉络
Chat Paper
正在生成论文摘要