rsdtlib: Remote sensing with deep-temporal data library
SOFTWAREX(2023)
摘要
For over a decade, satellite based remote sensing data have been intensively used for Deep Learning (DL) to help to identify Land Cover (LC) and Land Use (LU), and to detect urban and vegetation changes. Usually, these tasks are carried out with few samples or short and low-dimensional time series. In a recent study demonstrating urban change detection and monitoring, a windowed high - dimensional large time series (deep-temporal) was leveraged that not only considered a large amount of observations but also combined multiple modes for a higher temporal resolution. The software used in this approach for pre-processing, called rsdtlib, is described in the underlying work. It is made available to help others in the field of remote sensing to use this approach for Deep and Machine Learning (ML) solutions. The software is scalable to support a wide range of demands, including providing single observation samples, observation pairs, multiple modes, and the construction of windowed deep-temporal time series. Its output data is in a DL/ML training ready format and the software solution integrates well with existing remote sensing tools and services. The rsdtlib software is hosted on Github as an open source project to invite other researchers and practitioners in the remote sensing domain to utilize it.(c) 2023 Published by Elsevier B.V. This is an open access article under the CC BY license (http://creativecommons.org/licenses/by/4.0/).
更多查看译文
关键词
Remote sensing,Deep Learning,Machine Learning,Time series
AI 理解论文
溯源树
样例
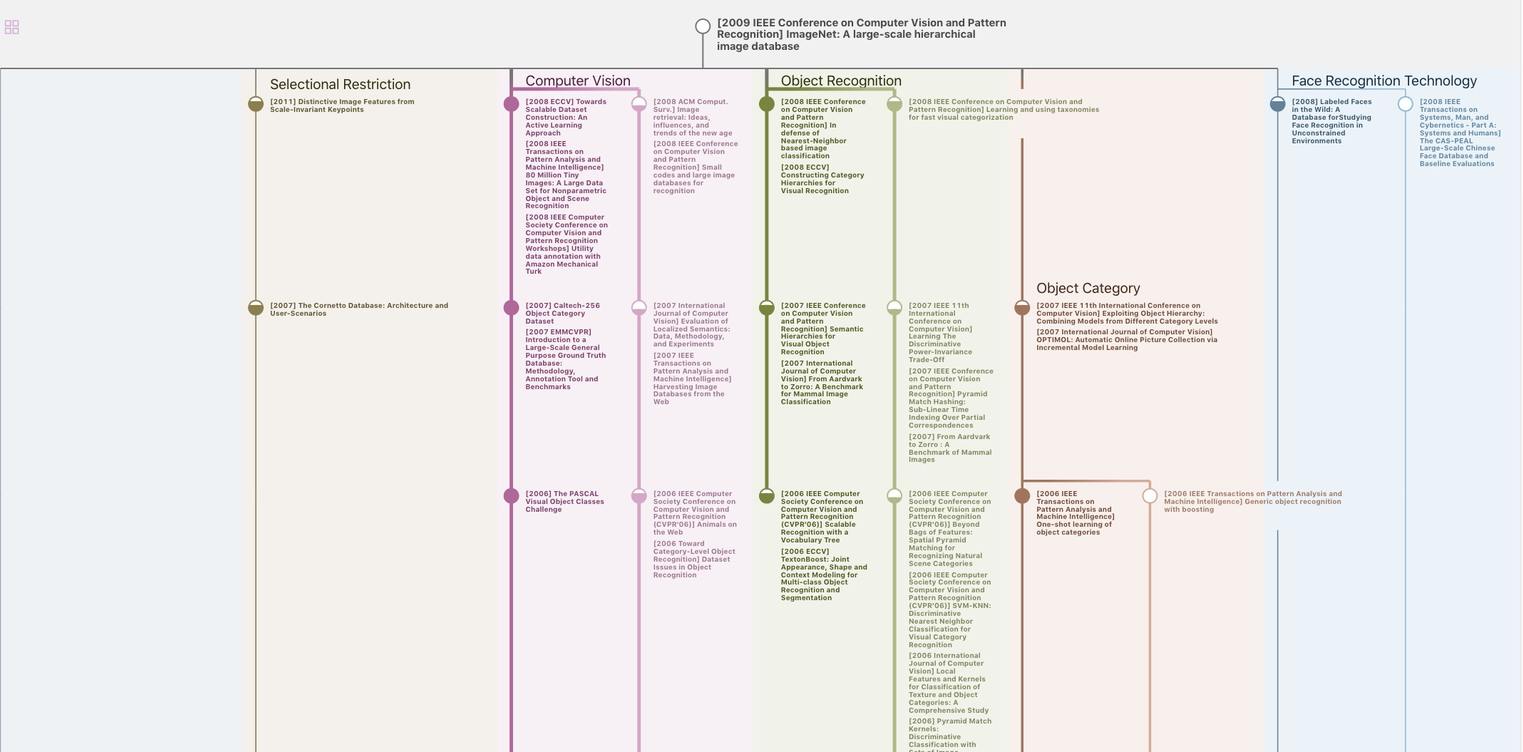
生成溯源树,研究论文发展脉络
Chat Paper
正在生成论文摘要