Hierarchical Transformer-based Query by Multiple Documents
PROCEEDINGS OF THE 2023 ACM SIGIR INTERNATIONAL CONFERENCE ON THE THEORY OF INFORMATION RETRIEVAL, ICTIR 2023(2023)
摘要
It is often difficult for users to form keywords to express their information needs, especially when they are not familiar with the domain of the articles of interest. Moreover, in some search scenarios, there is no explicit query for the search engine to work with. Query-By-Multiple-Documents (QBMD), in which the information needs are implicitly represented by a set of relevant documents addresses these retrieval scenarios. Unlike the keyword-based retrieval task, the query documents are treated as exemplars of a hidden query topic, but it is often the case that they can be relevant to multiple topics. In this paper, we present aHierarchical Interaction-based (HINT) bi-encoder retrieval architecture that encodes a set of query documents and retrieval documents separately for the QBMD task. We design a hierarchical attention mechanism that allows the model to 1) encode long sequences efficiently and 2) learn the interactions at low-level and high-level semantics (e.g., tokens and paragraphs) across multiple documents. With contextualized representations, the final scoring is calculated based on a stratified late interaction, which ensures each query document contributes equally to the matching against the candidate document. We build a large-scale, weakly supervised QBMD retrieval dataset based on Wikipedia for model training. We evaluate the proposed model on both Query-By-Single-Document (QBSD) and QBMD tasks. For QBSD, we use a benchmark dataset for legal case retrieval. For QBMD, we transform standard keyword-based retrieval datasets into the QBMD setting. Our experimental results show that HINT significantly outperforms all competitive baselines.
更多查看译文
关键词
Query by multiple documents,Hierarchical transformer,Neural re-ranking
AI 理解论文
溯源树
样例
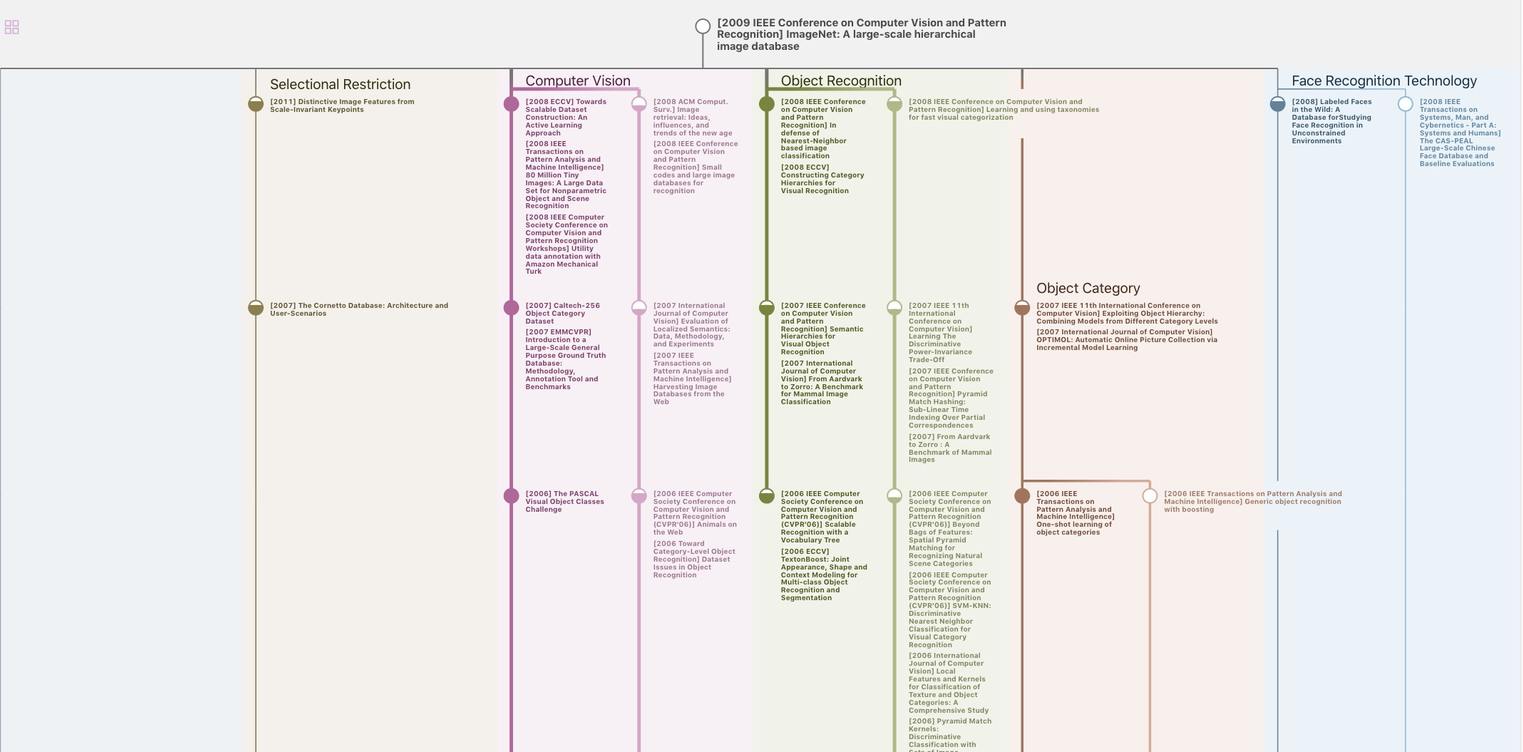
生成溯源树,研究论文发展脉络
Chat Paper
正在生成论文摘要