A practically feasible transfer learning method for deep-temporal urban change monitoring (vol 44, pg 5172, 2023)
INTERNATIONAL JOURNAL OF REMOTE SENSING(2023)
摘要
Neural networks have shown their potential to monitor urban changes with deep-temporal remote sensing data, which simultaneously considers a large number of observations within a given window. However, training these networks with supervision is a challenge due to the low availability of third-party sources with sufficient spatio-temporal resolution to label each window individually. To remedy this problem, we developed a novel approach utilizing transfer learning (TL) on a set of deep-temporal windows. We demonstrate that labelling of multiple windows simultaneously can be practically viable, even with a low amount of high spatial resolution third-party data. The overall process provides a trade-off between labour resources and the ability to train a network on existing systems, despite its intensive memory requirements. As a demonstration, an existing previously trained (pre-trained) network was used to transfer knowledge to a new target location. We demonstrate our method with combined Sentinel 1 and 2 observations for the area of Liege (Belgium) for the time period spanning 2017-2020. This is underpinned by our use of common metrics in machine learning and remote sensing, and in our discussion of selected examples. Three independent transfers of the same pre-trained model and their combination were carried out, all of which showed an improvement in terms of these metrics.
更多查看译文
关键词
feasible transfer,learning,change,deep-temporal
AI 理解论文
溯源树
样例
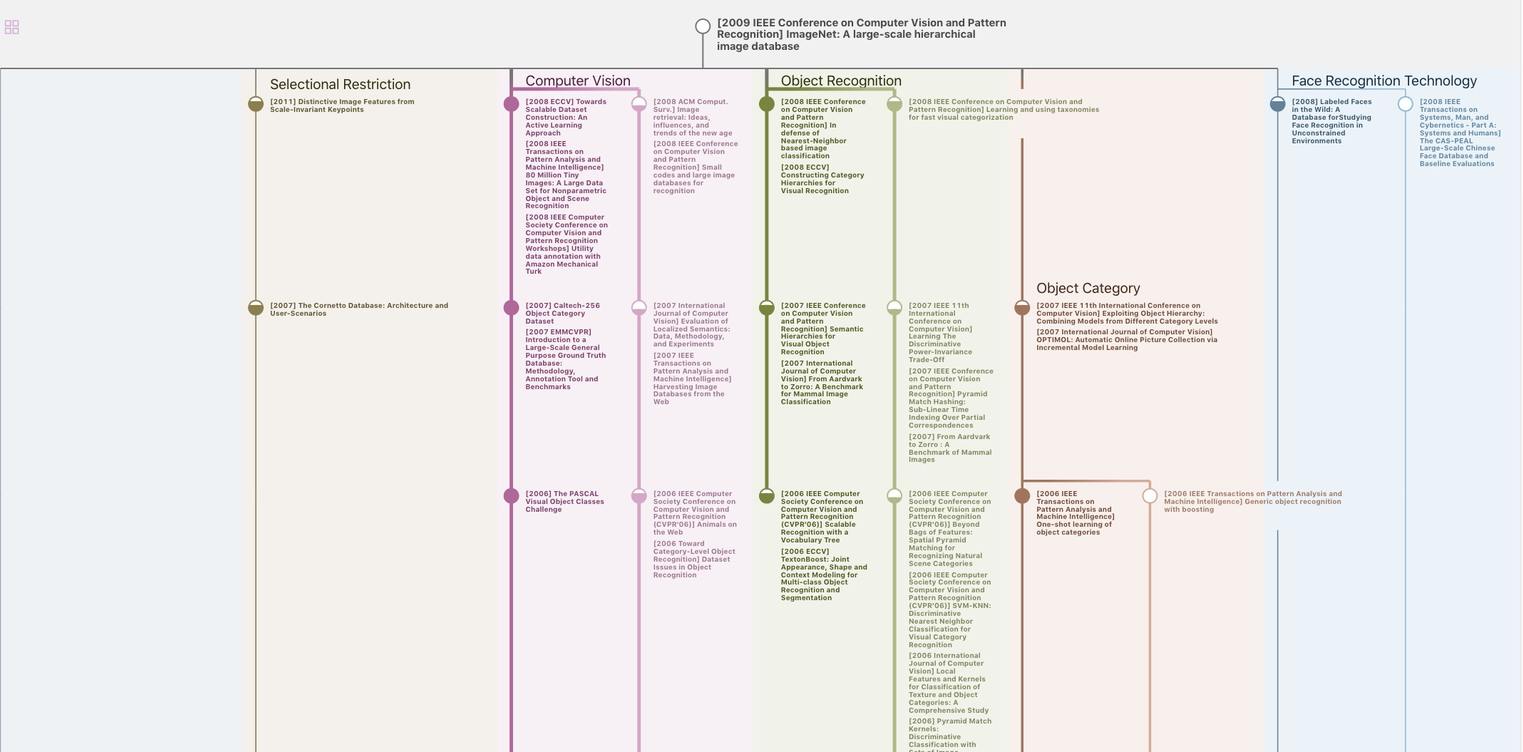
生成溯源树,研究论文发展脉络
Chat Paper
正在生成论文摘要